Deep-learning classification using convolutional neural network for evaluation of maxillary sinusitis on panoramic radiography
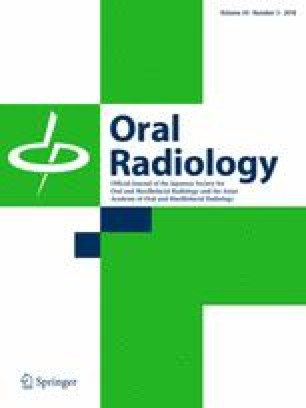
Abstract
Objectives
To
apply a deep-learning system for diagnosis of maxillary sinusitis on
panoramic radiography, and to clarify its diagnostic performance.
Methods
Training
data for 400 healthy and 400 inflamed maxillary sinuses were enhanced
to 6000 samples in each category by data augmentation. Image patches
were input into a deep-learning system, the learning process was
repeated for 200 epochs, and a learning model was created.
Newly-prepared testing image patches from 60 healthy and 60 inflamed
sinuses were input into the learning model, and the diagnostic
performance was calculated. Receiver-operating characteristic (ROC)
curves were drawn, and the area under the curve (AUC) values were
obtained. The results were compared with those of two experienced
radiologists and two dental residents.
Results
The
diagnostic performance of the deep-learning system for maxillary
sinusitis on panoramic radiographs was high, with accuracy of 87.5%,
sensitivity of 86.7%, specificity of 88.3%, and AUC of 0.875. These
values showed no significant differences compared with those of the
radiologists and were higher than those of the dental residents.
Conclusions
The
diagnostic performance of the deep-learning system for maxillary
sinusitis on panoramic radiographs was sufficiently high. Results from
the deep-learning system are expected to provide diagnostic support for
inexperienced dentists.
Comments