Dental caries diagnosis in digital radiographs using back-propagation neural network
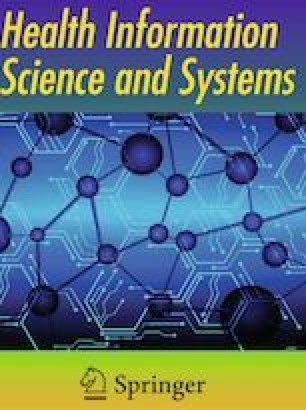
Abstract
Purpose
An
algorithm for diagnostic system with neural network is developed for
diagnosis of dental caries in digital radiographs. The diagnostic
performance of the designed system is evaluated.
Methods
The
diagnostic system comprises of Laplacian filtering, window based
adaptive threshold, morphological operations, statistical feature
extraction and back-propagation neural network. The back propagation
neural network used to classify a tooth surface as normal or having
dental caries. The 105 images derived from intra-oral digital
radiography, are used to train an artificial neural network with 10-fold
cross validation. The caries in these dental radiographs are annotated
by a dentist. The performance of the diagnostic algorithm is evaluated
and compared with baseline methods.
Results
The
system gives an accuracy of 97.1%, false positive (FP) rate of 2.8%,
receiver operating characteristic (ROC) area of 0.987 and precision
recall curve (PRC) area of 0.987 with learning rate of 0.4, momentum of
0.2 and 500 iterations with single hidden layer with 9 nodes.
Conclusions
This
study suggests that dental caries can be predicted more accurately with
back-propagation neural network. There is a need for improving the
system for classification of caries depth. More improved algorithms and
high quantity and high quality datasets may give still better tooth
decay detection in clinical dental practice.
Comments